Spark Introduction For Data Engineering
- Shamen Paris
- Jan 10, 2021
- 3 min read
Spark Introduction
Apache Spark is an open source, distributed process system used for large-scale data processing(Big Data).
Spark is fast because that Spark runs on memory(RAM), and that makes the processing much faster than on disk drives.
Spark x100 times faster than MapReduce.
Advantages of Spark
Speed - Run workloads 100x faster
Ease of Use - Write applications quickly in Java, Scala, Python, R, and SQL
Generality - Combine SQL, streaming, and complex analytics
Runs Everywhere - Spark runs on Hadoop, Apache Mesos, Kubernetes, standalone, or in the cloud. It can access diverse data sources.
Components
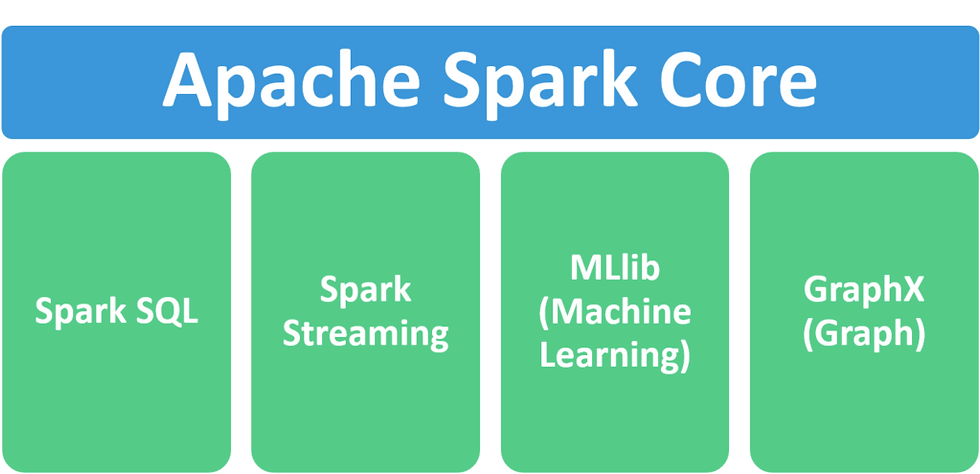
1.Apache Spark Core – Spark Core is the underlying general execution engine for the Spark platform that all other functionality is built upon. It provides in-memory computing and referencing datasets in external storage systems.
2.Spark SQL – Spark SQL is Apache Spark’s module for working with structured data. The interfaces offered by Spark SQL provides Spark with more information about the structure of both the data and the computation being performed.
3.Spark Streaming – This component allows Spark to process real-time streaming data. Data can be ingested from many sources like Kafka, Flume, and HDFS (Hadoop Distributed File System). Then the data can be processed using complex algorithms and pushed out to file systems, databases, and live dashboards.
4.MLlib (Machine Learning Library) – Apache Spark is equipped with a rich library known as MLlib. This library contains a wide array of machine learning algorithms- classification, regression, clustering, and collaborative filtering. It also includes other tools for constructing, evaluating, and tuning ML Pipelines. All these functionalities help Spark scale out across a cluster.
5.GraphX – Spark also comes with a library to manipulate graph databases and perform computations called GraphX. GraphX unifies ETL (Extract, Transform, and Load) process, exploratory analysis, and iterative graph computation within a single system.
Resilient Distributed Datasets (RDDs)
Data sharing is slow in MapReduce due to replication, serialization, and disk IO. Most of the Hadoop applications, they spend more than 90% of the time doing HDFS read-write operations.
Recognizing this problem, researchers developed a specialized framework called Apache Spark. The key idea of spark is Resilient Distributed Datasets (RDD)
Resilient Distributed Datasets (RDD) is a fundamental data structure of Spark. It is an immutable distributed collection of objects. Each dataset in RDD is divided into logical partitions, which may be computed on different nodes of the cluster. RDDs can contain any type of Python, Java, or Scala objects, including user-defined classes.
Formally, an RDD is a read-only, partitioned collection of records. RDDs can be created through deterministic operations on either data on stable storage or other RDDs. RDD is a fault-tolerant collection of elements that can be operated on in parallel.
There are two ways to create RDDs − parallelizing an existing collection in your driver program, or referencing a dataset in an external storage system, such as a shared file system, HDFS, HBase, or any data source offering a Hadoop Input Format
Iterative Operations on Spark RDD
The illustration given below shows the iterative operations on Spark RDD. It will store intermediate results in a distributed memory instead of Stable storage (Disk) and make the system faster.
Note − If the Distributed memory (RAM) is not sufficient to store intermediate results (State of the JOB), then it will store those results on the disk.
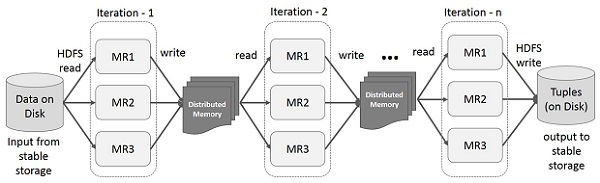
Interactive Operations on Spark RDD
This illustration shows interactive operations on Spark RDD. If different queries are run on the same set of data repeatedly, this particular data can be kept in memory for better execution times.
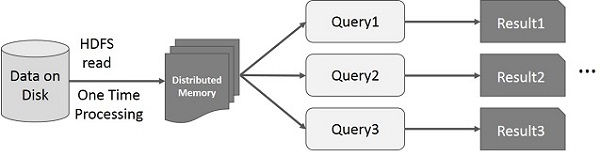
By default, each transformed RDD may be recomputed each time you run an action on it. However, you may also persist an RDD in memory, in which case Spark will keep the elements around on the cluster for much faster access, the next time you query it. There is also support for persisting RDDs on disk, or replicated across multiple nodes.
For more better understanding watch the below video:
I hope you got a good idea about spark
Good Luck!
Comments